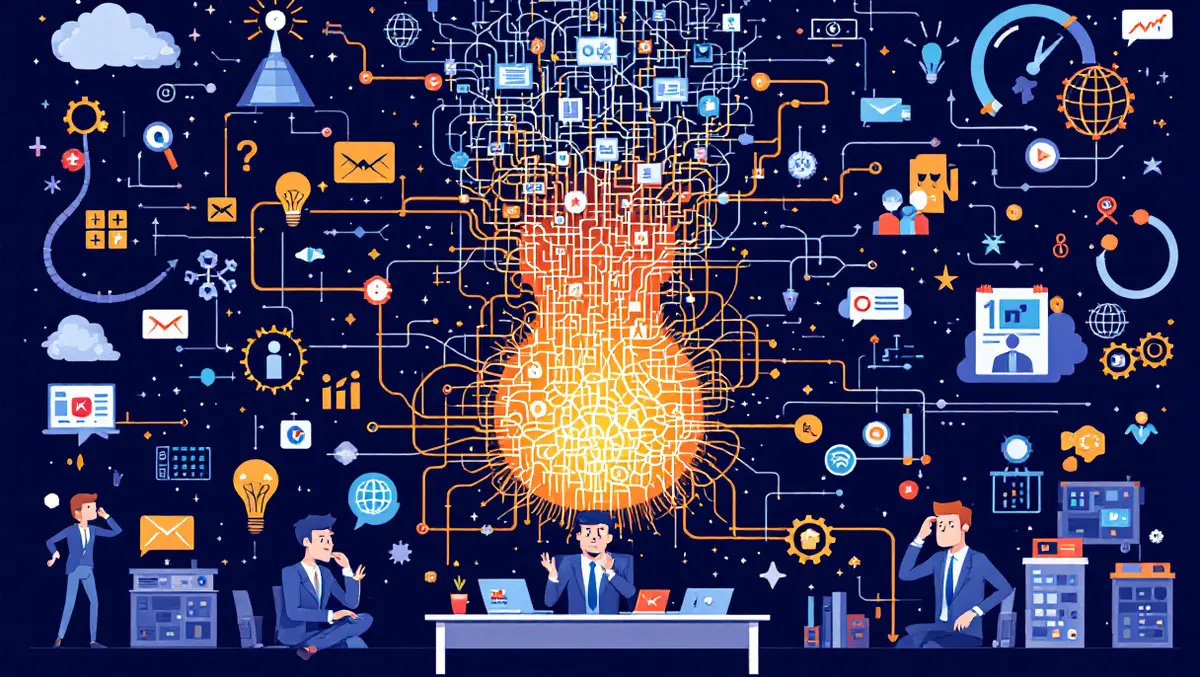
Data readiness issues lead to half of enterprise AI projects failing
Nearly half of enterprise artificial intelligence projects are failing due to poor data readiness, according to new research from Fivetran.
The survey, conducted by Redpoint Content, reveals a significant gap between organisations' AI ambitions and their ability to execute on these goals, citing complex integration and burdensome pipeline maintenance as main contributors to stalled progress.
Based on responses from 401 data leaders and professionals across technology, finance, healthcare, retail, and manufacturing sectors, the report finds that 42% of enterprises say more than half of their AI projects have experienced delays, underperformance, or failure because of data readiness issues.
This disconnect is evident despite 57% of organisations rating their data centralisation strategies as highly effective. The report points to a lack of fully centralised, real-time, and governed data as a primary reason for unsuccessful AI deployment.
The findings suggest that while enterprises may be pursuing bold AI and data centralisation strategies, many remain stuck managing complex infrastructure instead of delivering business value. As evidence, 67% of highly centralised enterprises say they allocate more than 80 percent of their engineering resources just to maintain data pipelines.
The operational impact of failed or delayed AI projects is notable. According to the survey, 38% of enterprises have experienced increased operational costs resulting from these failures. Lost revenue has also been reported, with 68% of organisations with less than half of their data centralised citing financial losses tied to underperforming AI efforts.
Customer satisfaction is also at stake, with reduced retention cited as the most common consequence of failed AI initiatives. Regulatory compliance poses an additional concern; 59% of respondents say it is their top challenge in managing data for AI.
The challenge of moving beyond pilot stages of AI is pronounced, as many enterprises are unable to efficiently prepare, integrate, or operationalise their data. The research highlights several contributing factors, including the prevalence of data silos and the need for real-time data access. Specifically, 41% of organisations say the absence of real-time data prevents AI models from delivering timely insights, while 29% report that data silos are significant obstacles to AI success.
Integration complexity is on the rise, with 74% of enterprises either managing or planning to manage more than 500 data sources. This amplifies the requirement for scalable and automated data integration solutions. According to the survey, nearly three-quarters of respondents acknowledge this growing complexity, which absorbs a high proportion of engineering resources and limits time spent on AI innovation.
Addressing these structural and operational bottlenecks has become a clear priority for many organisations. The report identifies that 65% of surveyed enterprises plan to invest in data integration tools as a primary strategy to enable AI over the coming period.
Regional and industry differences in AI readiness were also documented. Healthcare and retail sectors lead in this area, attributed to stronger automation and data integration strategies. Conversely, finance and manufacturing continue to face challenges stemming from outdated legacy systems and persistent integration issues.
From a geographic perspective, the Asia-Pacific region leads with an AI readiness score of 8.8 out of 10. The United States follows at 8.2, while the United Kingdom lags behind with a score of 6.0, which the report attributes to weak integration strategies and fragmented infrastructure.
"Nearly half of enterprises report delayed, underperforming, or failed AI projects despite bold strategies and major investment in AI and data centralisation. The disconnect is data that is not fully centralised, governed, or made available in real time for AI models. From integration bottlenecks to pipeline maintenance burdens, enterprises are stuck managing infrastructure instead of delivering business value through AI," the report states.
"Until these challenges are addressed, organisations will continue to struggle with AI performance and fail to unlock the full value of their investments," the survey report adds.
The survey was conducted in the first quarter of 2025, with responses collected from enterprises ranging from 500 to more than 5,000 employees across multiple regions including the United States, United Kingdom, Europe, the Middle East, Africa and the Asia-Pacific.